Generative AI: Pushing Boundaries of Innovation
- Thought Leadership
- February 8, 2024
Over the years, advancements in deep learning, particularly with GANs, have significantly enhanced the accuracy and diversity of generated content. Generative AI, powered by innovative technologies like ChatGPT and GANs, is reshaping the way we create and interact with content. This blog explores the evolution of Generative AI, from its historical roots to its current capabilities and future prospects.
Innovation in the realm of AI and ML has been relentless, continually redefining what’s possible as the technology gets more and more robust. Among the manifold branches of AI, Generative AI has taken center stage in recent times due to tools like ChatGPT, Jasper, Synthesia, etc. that have revolutionized how we create, design, interact, and consume information.
What is Generative AI?
Generative AI is AI models or algorithms that generate content, such as text, photos, videos, code, data, or 3D renderings, from the vast amounts of data they are trained on. The models produce new content based on the data they have been trained on, allowing them to deliver intelligent responses. But the question has always been about how accurate they are. The responses are solely based on how strong the training data is.
Well, sometime back, I watched a video about how David Choe (an American artist, musician, and actor) fired his attorney for charging him $600 per consultation to answer his legal queries. The answers provided by his attorney were the same as the answers of ChatGPT. This may sound very surprising but at the same time, it amazes me how accurate these tools are.
History of Generative AI
You may wonder how generative AI may have developed over the last few years. However, generative AI didn’t emerge in recent times, its history dates back to the 1950s-60s when it was first introduced in chatbots.
Some of the first generative models were known as Hidden Markov Models (HMMs) and Gaussian Mixture Models (GMMs) that were developed during the 50s. For HMMs, one of the first applications was speech recognition. The productivity of generative models, though, was significantly boosted only after the rise of deep learning.
It was not until 2014, with the introduction of Generative Adversarial Networks (GANs), a type of machine learning algorithm that generative AI could create convincing images, videos, and audio of people with accuracy. Over the years as the datasets get larger, the accuracy has also improved for generative AI widening the realm of possibilities.
How Generative AI has Progressed
The progress of Generative AI can be attributed to advancements in deep learning. Let’s see how it has influenced various facets of content development.
Language Generation
Generative AI Models like ChatGPT have demonstrated remarkable capabilities in generating intelligent and relevant near-human responses. They have been employed for various natural language processing (NLP) activities, such as language translation, summarization, and creating intelligently written content.
Text to Image Generation
Generative Adversarial Networks (GANs) have been widely used for image synthesis (generating artificial images). Models like StyleGAN and BigGAN have shown the ability to generate high-quality and diverse images by just typing in a short description of what you want to see. However, this has also raised a lot of concerns due to the deepfakes that are being generated and shared.
Audio Generation
A lot of progress has been made in generating realistic and expressive audio, including speech synthesis and music composition. WaveGAN and Tacotron are examples of models that have shown improvements in generating human-like audio.
Many tools are also available that turn written text into a voiceover, making it easier for content creators to deliver the content faster. However, it may lack human emotion so it may not be useful for all purposes.
Video Synthesis
Advancements in video generation involve the synthesis of realistic and diverse video content. Deepfake technology, despite its controversies, is a great example of generative AI applied to video. allowing for realistic face-swapping and scene generation. This may be beneficial for motion pictures and documentaries.
Interactive and Conditional Generation
Generative models have become more interactive and capable of conditional generation. Conditional GANs and models like DALL-E by OpenAI showcase the ability to generate content based on specific inputs or constraints.
Fine-Tuning and Transfer Learning
AI has made many strides in fine-tuning trained AI models for specific tasks, reducing the need for extensive training data. This has led to more efficient and effective use of generative models despite their applications.
Ethical and Safety Considerations
As generative AI continues to grow, there is increased attention on ethical considerations and the potential misuse of these technologies. There needs to be proper guidelines and policies that minimize misuse. Many organizations are working on guidelines and policies to ensure responsible development and deployment.
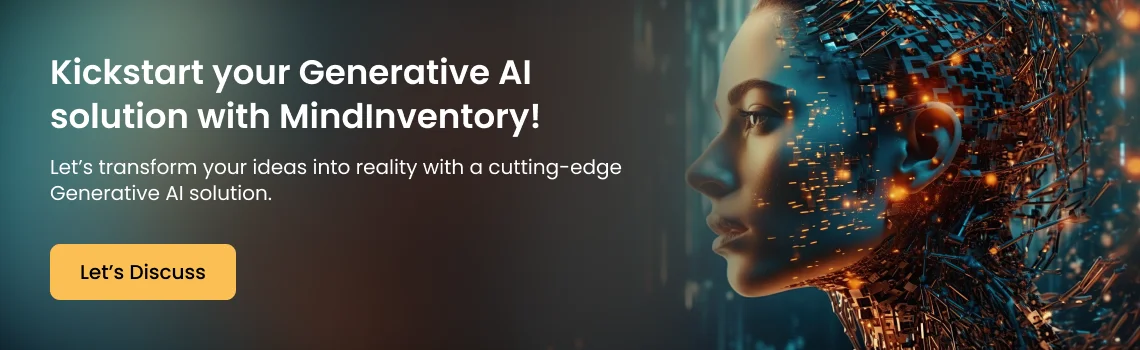
The Future of Generative AI
To be honest, we are still in the nascent stages of exploring what generative AI can accomplish. It is a fact that generative AI has not come to the level to completely mimic human behavior, especially the emotional aspect.
This may take some time, however, it will get more personalized and become more human-like in the future. We can definitely see the role of Gen AI seeping into all industry verticals and becoming an integral part of the day-to-day operations of many businesses.
On the software applications front, we will definitely see the development cycles get shorter and the time to market getting accelerated. This will be because developers will not have to develop from scratch and designers do not have to design from scratch. Even when it comes to video games, it takes a lot of time to create environments, and with generative AI you will be able to generate these surrounding environments faster, which also accelerates time to market.
The Gen AI of the future will be able to understand factors such as human psychology and their creativity process in greater depth, enabling them to create content that’s deeper and more engaging. OpenAI’s GPT4, Mistral, Meta’s Llama 2, and others have all made considerable advancements in large language models (or Multimodal capabilities). These models have evolved from the traditional single-mode functions which generated single outputs.
However, in the future, we may see language models incorporating and mixing diverse data types such as images, language, and audio. Because of these kinds of transitions, AI will become much more dynamic and cohesive.
For the future, the possibilities are endless and generative AI can be a disruptor in many industries. Get in touch with us to know how MindInventory can help you leverage AI and ML to deliver exceptional products and services.