How AI is Transforming Banking: Benefits, Use Cases & Future Trends
- AI/ML
- June 14, 2024
The banking sector is no stranger to innovation, but Artificial Intelligence (AI) is revolutionizing the industry like never before. From detecting fraudulent transactions to offering hyperpersonalized financial advice, AI is transforming how banks operate and interact with customers.
According to McKinsey, AI could generate $1 trillion in annual value for global banking proof that this technology isn’t just a trend but a strategic imperative.
But what makes AI/ML development services indispensable for modern banking? Simple: speed, accuracy, and scalability. While traditional methods rely on manual processes, AI analyzes vast datasets in real time, predicts risks, automates repetitive tasks, and even understands human emotions through Natural Language Processing (NLP). Whether you’re a customer enjoying instant loan approvals or a banker spotting anomalies in milliseconds, AI powered baking applications developed by hiring dedicated AI developers are driving efficiency.
Fun Fact: Over 80% of banks now use AI powered chatbots for customer service and that’s just the beginning.
Key Applications of AI in Banking
Artificial Intelligence is reshaping banking across every function, from customer service to risk management. Machine learning (ML), natural language processing (NLP), and robotic process automation (RPA) power these innovations, enabling banks to operate faster, smarter, and more securely. Here’s a breakdown of AI’s most impactful applications:
1. Fraud Detection & Prevention leverages AI to monitor transactions in real time, identifying suspicious patterns like logins or unusual spending spikes. Mastercard’s Decision Intelligence tool, for example, analyzes over a million data points per transaction to reduce false declines by 50% (IBM).
Key impact: AI cuts fraud losses by 30–50% while minimizing false alarms.
2. Chatbots & Virtual Assistants, powered by NLP and synthetic training data, handle routine inquiries (balance checks, payment reminders) with human like precision. Bank of America’s Erica and HSBC’s Amy resolve 80% of queries without human intervention, slashing customer service costs by 30% (Accenture).
Key impact: 24/7 support with 90% customer satisfaction rates.
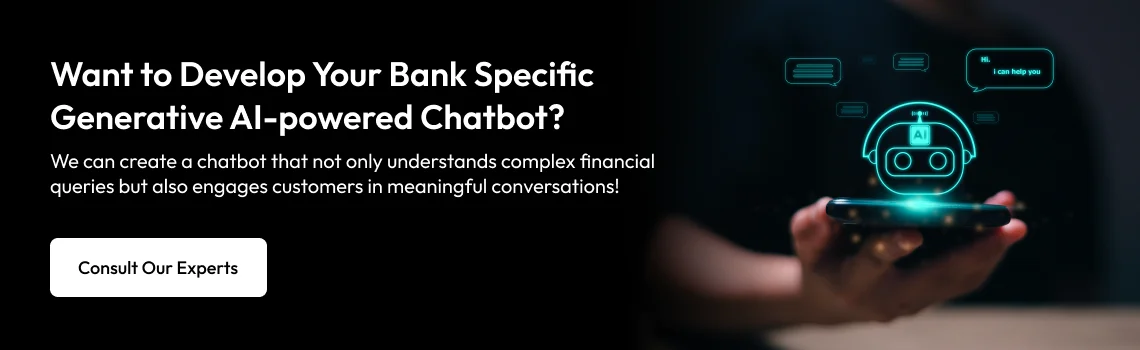
3. Personalized Banking uses AI to analyze spending habits and offer tailored advice, like savings goals or investment tips. Apps like Cleo even use humor to engage users, with 72% of customers preferring banks that customize services (Evergage).
Key impact: Boosts customer retention and cross selling by 20%.
4. Credit Scoring & Loan Approvals now incorporate alternative data (e.g., rent payments, social activity) via ML models. ZestFinance approves loans for thin file borrowers 40% more accurately, while reducing approval times to under 5 minutes (McKinsey).
Key impact: Expands financial inclusion with 20% lower default rates.
5. Risk Management & Compliance (RegTech) automates tedious tasks like anti money laundering (AML) checks. JPMorgan’s COiN reviews 12,000 contracts in seconds work that previously took 360,000 human hours.
Key impact: Reduces compliance costs by 50–70% (Deloitte).
6. Process Automation (RPA) tackles back office tasks like data entry and KYC verification. Deutsche Bank’s RPA bots handle 65% of trade finance operations, saving 200+ hours monthly per employee (EY).
Key impact: Cuts operational costs by 25–40%.
Benefits of AI in Banking
The adoption of Artificial Intelligence (AI) in banking is no longer optional—it’s a necessity. Financial institutions leveraging AI gain a competitive edge through enhanced security, superior customer experiences, cost savings, and faster decision making.
As highlighted in this AI in Fintech article, the integration of intelligent technologies is transforming the financial sector. Here’s are the benefits of AI in banking:
1. Enhanced Security & Reduced Fraud
AI powered fraud detection systems analyze millions of transactions per second, identifying suspicious activity in real time. Unlike traditional rule based systems, machine learning (ML) adapts to new fraud patterns, reducing false positives and stopping cybercriminals before they strike. To build such intelligent solutions, many organizations choose to hire ML developers who can tailor models to evolving threats.
- Impact: Banks using AI for fraud prevention report 30–50% fewer losses (IBM)
- Example: AI driven behavioral biometrics can detect account takeovers by tracking typing speed, mouse movements, and login habits.
2. Improved Customer Experience (Hyper Personalization)
Customers today expect tailored financial solutions, and AI makes it possible. By analyzing transaction history, spending habits, and even social signals, AI delivers personalized recommendations from savings tips to investment opportunities.
- Impact: 72% of customers prefer banks that offer customized services (Evergage).
- Example: AI powered chatbots like Capital One’s Eno proactively alert users about unusual charges or subscription price hikes.
3. Cost Savings & Operational Efficiency
Manual banking processes are time consuming and expensive. AI automates repetitive tasks such as data entry, compliance checks, and customer onboarding freeing employees to focus on high value work.
- Impact: Banks using Robotic Process Automation (RPA) save 25–40% in operational costs (EY).
- Example: Deutsche Bank automates 65% of trade finance operations with AI, reducing errors and processing times.
4. Faster, Data Driven Decision Making
AI crunches vast datasets in real time, helping banks make smarter, faster decisions—whether approving loans, predicting market trends, or optimizing investment portfolios.
- Impact: AI powered credit scoring approves loans 5x faster with 20% lower default rates (McKinsey).
- Example: JPMorgan Chase’s COiN analyzes 12,000 contracts in seconds, a task that once took 360,000 human hours.
Real World Examples of AI in Banking
Leading banks are already harnessing AI to optimize operations, enhance security, and redefine customer service. Here are some standout implementations:
1. JPMorgan Chase: COiN for Contract Analysis
Uses NLP and ML to review 12,000 legal documents in seconds, a task that previously took 360,000 human hours annually.
Impact: Saves millions in legal costs and reduces human error.
2. HSBC: AI Powered Anti Money Laundering (AML)
Deploys machine learning to detect suspicious transactions with 99% accuracy, far surpassing traditional rule based systems.
Impact: Reduced false alerts by 60%, accelerating compliance workflows (HSBC Case Study).
3. Capital One: Eno, the AI Driven Assistant
A predictive chatbot that monitors accounts for fraud, subscription hikes, and overdrafts, sending proactive alerts.
Impact: Handles 7+ million customer interactions monthly with 90% resolution rates (Capital One).
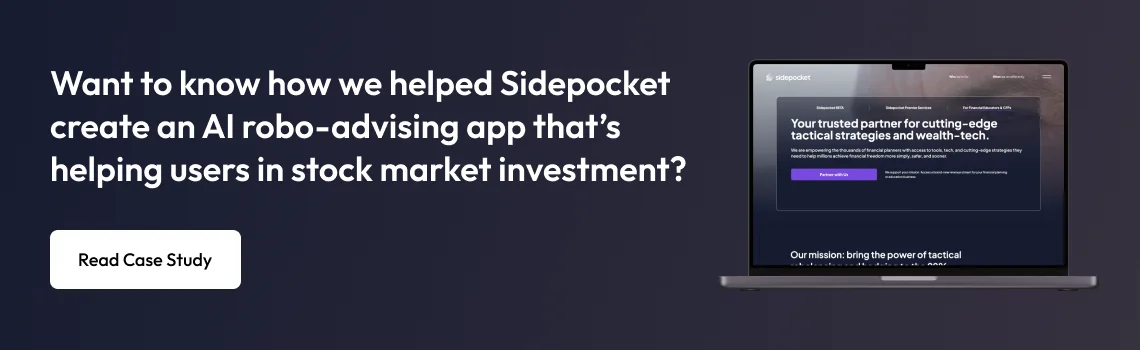
Challenges & Risks of AI Adoption in Banking
While AI offers transformative benefits for banks, its adoption comes with significant challenges and risks that financial institutions must address.
1. Data Privacy & Security Concerns
Banks handle sensitive customer data, making them prime targets for cyberattacks. AI systems, if not properly secured, can be vulnerable to data breaches, adversarial attacks, or misuse of personal information. Strict GDPR and CCPA compliance is essential, but balancing security with AI driven personalization remains a challenge whether implementing computer vision for identity verification or other AI solutions.
2. High Implementation Costs
Deploying AI requires massive investments in infrastructure, talent, and ongoing maintenance. Many banks, especially smaller ones, struggle with the ROI justification—despite long term savings, upfront costs can be prohibitive.
3. Bias in AI Algorithms
If trained on historically biased data, AI models can perpetuate discrimination in loan approvals, credit scoring, or hiring. For example, an AI system might unfairly deny loans to marginalized groups due to flawed training data.
4. Regulatory Hurdles
The banking sector is highly regulated, and AI adoption often outpaces legal frameworks. Regulators are still catching up on AI transparency, explainability, and accountability, creating compliance uncertainties.
Future Trends in AI for Banking (2025 and Beyond)
The future of AI in banking is even more dynamic, with emerging technologies set to redefine financial services.
1. Hyper Personalization with Generative AI
Banks will use ChatGPT like AI to offer real time, conversational financial advice, customized investment strategies, and even AI generated financial reports. Imagine an AI assistant that predicts cash flow issues before they happen.
2. Voice Activated Banking
Voice assistants like Alexa, Siri, and Google Assistant will enable seamless voice based transactions, balance checks, and fraud alerts. HSBC and Wells Fargo are already testing voice enabled payments.
3. Blockchain + AI for Smarter Contracts
AI will enhance smart contracts by automating compliance checks, detecting fraud, and optimizing settlements. JPMorgan’s Onyx blockchain is a prime example of this fusion.
4. Central Bank Digital Currencies (CBDCs) & AI
As more countries explore CBDCs as part of their digital transformation in banking initiatives, AI will play a key role in preventing money laundering, optimizing monetary policy, and ensuring transaction efficiency. The European Central Bank (ECB) is already testing AI driven CBDC models.
Why Choose MindInventory for AI in Banking?
The banking sector’s rapid adoption of AI demands cutting edge expertise, seamless integration, and scalable solutions—and that’s where MindInventory excels. With years of experience in AI/ML development for financial services, we empower banks to harness predictive analytics, fraud detection, hyper personalization, and process automation with unmatched precision.
Our team of seasoned AI engineers and data scientists for hire specializes in building bespoke AI solutions, from Generative AI powered chatbots to blockchain enhanced fraud prevention systems, ensuring your bank stays ahead in efficiency, security, and customer satisfaction.
What sets us apart? Proven success in deploying AI for global financial institutions, adherence to strict compliance standards (GDPR, PCI DSS), and a commitment to ethical, bias free AI models. Whether you’re looking to automate loan approvals, enhance AML checks, or launch a voice activated banking assistant, MindInventory delivers end to end AI development tailored to your goals.
Ready to transform your banking operations with AI? Let’s turn your vision into reality—share your ideas with us today!
FAQs on AI in Banking
AI is being deployed across multiple banking functions, including fraud detection through real time transaction monitoring, customer service via intelligent chatbots like Bank of America’s Erica, personalized financial recommendations using robo advisors, and automated loan approvals with alternative credit scoring. Additionally, AI streamlines compliance through RegTech solutions and enhances back office efficiency with robotic process automation (RPA).
AI offers banks significant advantages, such as improved security through advanced fraud detection, enhanced customer experiences via hyper personalized services, cost reductions by automating manual processes, and faster decision making through data driven insights. These benefits collectively lead to higher operational efficiency and increased customer satisfaction.
The future of AI in banking includes hyper personalized services powered by generative AI (like ChatGPT for financial advice), voice activated banking through smart assistants, blockchain integrated AI for secure smart contracts, and AI driven central bank digital currencies (CBDCs) for optimized monetary policies. These innovations will redefine customer interactions and operational efficiency.
AI automates compliance tasks such as anti money laundering (AML) checks, suspicious activity reporting, and audit trail generation. Tools like JPMorgan’s COiN can review thousands of legal documents in seconds, cutting compliance costs by 50–70% while minimizing human error.
Begin by identifying high impact use cases (e.g., fraud detection, chatbots), then partner with experienced AI developers or fintech providers. Pilot programs, staff training, and phased rollouts help ensure smooth integration while maintaining regulatory compliance.