Predictive Analytics in Insurance: Top Benefits, Use Cases, Real World Examples
- AI/ML
- May 19, 2025
Predictive analytics in insurance is reshaping how insurers operate by turning vast data into actionable insights to forecast risks, improve customer experiences, and more. But how does it work, and what real-world value does it offer? This blog breaks it all down, from how predictive analytics works in insurance to its key use cases, applications, implementation steps, challenges, and solutions.
Predictive analytics in insurance processes has been in use by insurers for decades, long before it became a buzzword. The only difference we see is the evolution of predictive models. Then, insurers were employing actuarial tables and manual linear regression and statistical calculations. Now, they are leveraging advanced predictive systems powered by machine learning, real-time behavioral insights, and telematics data to predict scenarios and decisions.
This evolution in predictive analytics for insurance operations is working in favor of insurers. The Willis Towers Watson survey on predictive analytics in insurance revealed that around 67% of insurers have seen a reduction in policy issuance and underwriting expenses. Plus, 60% of insurers have seen an increase in sales and profitability post-adopting advanced predictive analytics. Moreover, 83% of insurers also think that predictive analytics plays a key role in underwriting processes.
So, how is predictive analytics used in insurance? Well, that’s what this blog covers – from its benefits and applications to what the future holds.
Is your insurance firm stuck in a pool of data and looking to build a solution to craft data-driven strategies for better business benefits? Check out our Data Science Services! |
What is Predictive Analytics in Insurance?
Predictive analytics in insurance stands for a solution that leverages massive data and statistical modeling to forecast possibilities (future events and outcomes). Models used in it understand customer behavior to anticipate potential risks and further help insurers to make informed decisions to improve business operations and profitability.
Definitely, there’s a key role for predictive analytics in health insurance, life insurance, and auto insurance areas. However, at the latest, it has become a critical tool for P&C carriers (Property & Casualty Insurance providers). Well, that’s just the trend about predictive analytics across insurance types.
To implement predictive analytics, it’s a must for businesses to implement a digital technological transformation strategy, supporting data at a massive scale in an organized manner. Their data ecosystem (data pipelines + scalable data lakes) connected with AI models plays a key role in achieving human-centric insights.
There, our data engineering services can help you better prepare data and set up architecture for predictive analytics.
How Predictive Analytics Works in Insurance
Predictive analytics in insurance works by using historical and real-time data to forecast future outcomes, behaviors, or risks, enabling insurers to make smarter, faster decisions.
But what’s the magic spell for that output? Well, it’s the step-by-step process the predictive model performs on insurance data to provide insights, which includes:
1. Data Ingestion from Diverse Sources
Insurers gather vast volumes of both structured and unstructured data from multiple sources, such as customer demographics, claims history, application forms, medical and credit reports, vehicle telematics, social media activity, and digital behavior footprints. With the help of data pipelines, it ingest data from various sources to the predictive model.
2. Data Processing and Feature Engineering
The collected data is cleaned, standardized, and transformed to improve accuracy, consistency, and relevance. Feature engineering then helps convert raw inputs into meaningful variables that enhance model performance.
3. Pattern Recognition and Model Application
Predictive models built with statistical methods and machine learning models help insurers to identify correlations and patterns in the data. Then, these recognized patterns are used to predict outcomes, such as the likelihood of a claim being fraudulent or customer churn.
4. Scoring and Segmentation
Each claim, policy, or customer is assigned a predictive score or risk rating. These outputs are used to create meaningful segments, such as high-risk vs. low-risk drivers, claims eligible for fast-track vs. manual review, or customers likely to renew vs. churn.
5. Decision-Making and Action
Business decision-making and action take place in the form of underwriting rules adjusting policy pricing, escalation of flagged claims for deeper investigation, and boosts in marketing and retention campaigns for at-risk customers.
6. Feedback Loop and Model Improvement
Based on the feedback loop and model improvement, the predictive model continuously improves to achieve greater accuracy over time.
In short, predictive analytics works as a closed-loop system within insurance, in which historical and real-time data flow through analytical models that deliver predictions.
Top 8 Benefits of Predictive Analytics in Insurance
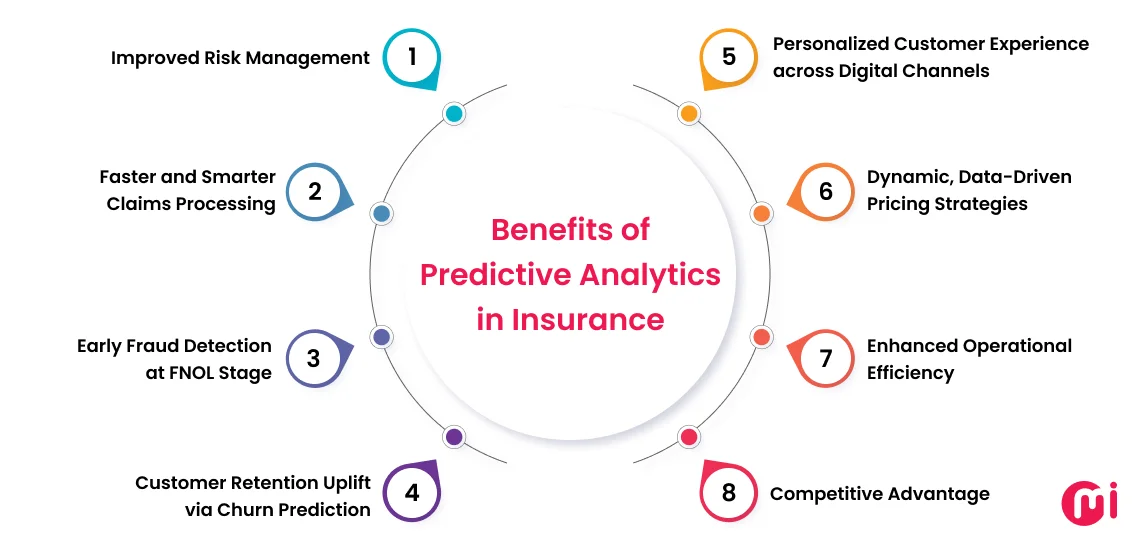
The use of predictive analytics in insurance processes enables you to access data to make strategies for customer retention, risk management, and customer experience. You can also use those data-driven insights to detect fraud early on to avoid further losses.
Below mentioned are the most alluring benefits of predictive analytics in insurance that includes:
1. Improved Risk Management
The integration of predictive analytics within insurance platforms helps insurers assess historical claims, behaviors, and external variables. This data analysis provides them access to quantify risk at an individual and portfolio level. Further, integrated AI models with predictive models help insurance underwriters make smarter, data-backed decisions to target more profitable risk pools and fewer unexpected losses.
2. Faster and Smarter Claims Processing
Modern predictive models integrated with AI capabilities enable insurers to assess claim severity, authenticity, and settlement potential immediately after submission. Based on this analysis, insurers can make decisions quickly, minimize manual interventions, and accelerate straight-through processing (STP) for low-risk claims. This enablement then improves turnaround times and customer satisfaction.
3. Early Fraud Detection at FNOL Stage
The First Notice of Loss (FNOL) stage is the first formal report about a potential claim that a policyholder does to their insurance company. At this stage, predictive analytics can be helpful to insurers to flag fraudulent claims by assessing anomalies in claimant behavior, policy history, or loss patterns.
Just like a medical drill in an emergency situation, insurers do follow triage. Thanks to the flag information through predictive analytics, insurers can triage suspicious claims early, avoid investigative overhead, and thus reduce financial losses.
4. Customer Retention Uplift via Churn Prediction
The use of predictive analytics in insurance platforms enables insurance companies to monitor behavioral patterns, payment history, and engagement trends to identify high risk of churn. This data-driven information helps insurance executives to proactively engage with at-risk customers by targeting personalized retention campaigns.
This data-driven decision-making helps insurers to improve customer loyalty towards your insurance company and, thus, customer lifetime value.
5. Personalized Customer Experience across Digital Channels
When you integrate predictive analytics into your insurance platform, you can also give it access to each and every customer interaction with your platform. This enables it to assess customer behavior, interactions, and thus preferences to predict their future needs.
With that, you can also fine-tune it to enable dynamic personalization for customers and offer them policy recommendations, proactive risk advice, and relevant add-ons across web, app, and chatbot interactions in a way they need.
6. Dynamic, Data-Driven Pricing Strategies
Instead of relying on broad risk categories, predictive models allow insurers to price premiums dynamically based on a wide range of data points, which include telematics, lifestyle behavior, and credit history. This enables you to set more competitive and fair pricing for policy for that particular customer/prospect, either through usage-based or pay-as-you-go insurance models.
7. Enhanced Operational Efficiency
As predictive analytics does the groundwork for insurers, like identifying potential customers, at-risk customers, fraudulent claims, and more, they get to focus on higher-value tasks. This enablement helps them improve productivity and get free from unnecessary operational overhead, which ultimately leads them to enhanced operational efficiency.
8. Competitive Advantage
Predictive analytics in insurance platforms enables insurers to stay ahead of the curve with faster innovation cycles, sharper risk insights, and more agile customer strategies. This fosters differentiation in crowded markets, enhances brand trust, and enables quicker adaptation to emerging risks and regulatory changes.
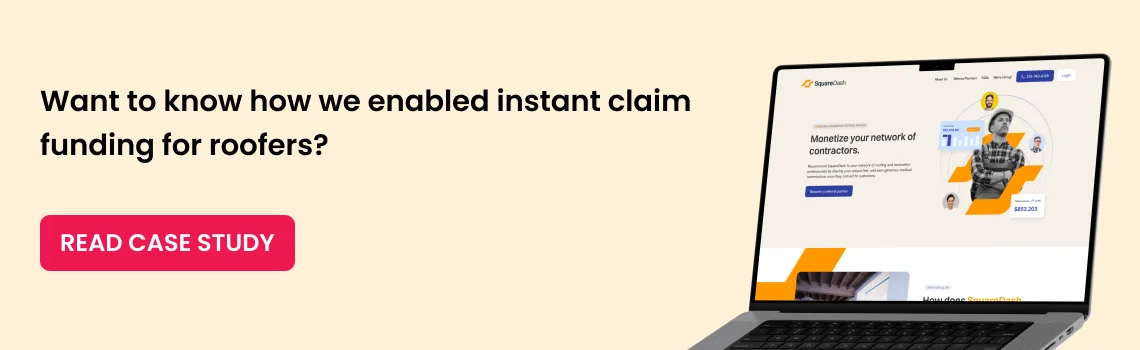
7 Key Use Cases of Predictive Analytics in Insurance
Among many digital trends in insurance, predictive analytics offers several use cases, such as identifying at-risk customers, detecting fraud, optimizing policy underwriting, improving customer retention, and more. Below are key use cases that insurance businesses should focus on by integrating predictive analytics into their operations:
1. Identifying High-Risk Drivers for Auto Insurance
You can use predictive analytics in auto insurance processes to leverage telematics data, driving behavior, past claims, and demographic details to score driver risk levels. By assessing all these key insights gathered from an auto insurance app and other sources, insurers can personalize premiums and take proactive steps to reduce potential loss exposure.
2. Predicting Health Conditions in Life & Health Insurance
The use of predictive analytics in life and health insurance enables insurers to leverage data from various sources to predict chronic disease risks or early onset conditions. Here, sources can be medical histories, lifestyle data, wearable device inputs, and social determinants of health.
Through these data insights, insurers can optimize underwriting and offer preventive care-based insurance to potential customers to make better business profits while contributing to the healthcare industry.
3. Reducing Fraud & Forecasting Natural Disaster Impact in Property Insurance Coverage
In the category of property insurance, the majority of claims are related to repair work done due to property age or any natural calamities. In this finding, the difference between genuine and fraudulent claims becomes important. There, predictive analytics plays a key role in the property & casualty (P&C) segment.
To optimize policy coverage, reinsurance, and reserves for natural disaster impact on property, predictive analytics help to provide insights on geographic data, weather models, and historical disaster trends. These help to predict which properties are likely to be affected by events like floods, wildfires, or hurricanes.
To detect fraud related to property insurance claims, it helps to flag fraudulent claims by analyzing customers’ historical claim patterns, repair estimates, and claimant behaviors.
Also explore the role of AI in real estate to know more use cases.
4. Predicting Customer Lifetime Value (CLV)
For any insurance business to succeed, it’s paramount to optimize resources and build customer loyalty. Predictive analytics can help significantly to predict customer lifetime value by analyzing policyholder behavior, engagement history, and renewal trends.
5. CAT Event Risk Modeling and Coverage Calibration
Here, CAT stands for catastrophic event, which is a risk model that uses predictive analytics to simulate the frequency and severity of large-scale events. It helps insurers calibrate their coverage offerings, determine capital requirements, and improve underwriting resilience.
6. Enhancing Cross-Sell and Upsell Strategies
In an insurance business, every sales agent must have strategies to cross-sell or upsell their services. Traditionally, they used to rely on their existing conversation or association with customers to plan their next move for cross-selling or upselling services. Their traditional strategies include identifying related or complementary products for existing clients and offering them higher-tier or more comprehensive coverage options.
With the use of predictive analytics in insurance products, the process has revolutionized into being more data-centric, helping insurers calibrate their coverage offerings, determine capital requirements, and improve underwriting efforts.
Top 5 Applications of Predictive Analytics in Insurance
Predictive analytics in insurance offers a wide range of applications, including proactive risk finding and mitigation, customer experience personalization, optimizing underwriting, and more.
With the digital trend evolving forYou can measure the ROI of predictive analytics in insurance by considering three main metrics. Metric 1: Time-to-decision reduction; Metric 2: Fraud detection false positives/negatives ratio; Metric 3: Employee productivity gains. the insurance industry, predictive analytics has also evolved as a great trend that’s benefiting the insurance industry in decision-making and strategies.
So, how is predictive analytics used in insurance operations? Well, below we’ve answered by mentioning top 5 predictive analytics applications in insurance operations answer that:
1. Hyper-Accurate Risk Assessment & Mitigation
Predictive models integrated in insurance applications have access to various data sources and types of data, such as customer demographics, behavior, and historical claims. Analyzing that data, predictive analytics enables insurers to assess risks with greater precision and mitigate them before they even occur.
These data insights lead insurers to make more accurate underwriting decisions and premium pricing, ensuring that policies are appropriately matched to individual risk profiles.
2. Personalized Customer Experiences
With predictive analytics, insurers can know what their customers like, what they’re planning to buy next, their challenges, and how and for what purpose they raise insurance claims.
All these data enable insurers to personalize policy recommendations, communication, and services. This personalized customer experience leads them to achieve improved customer engagement and loyalty.
3. Real-Time Fraud Detection
Insurance fraud is a significant global issue, costing provider companies billions annually. This raises a serious concern for implementing fraud detection solutions. That’s where predictive analytics comes to the rescue.
Predictive analytics helps to analyze all claims in real-time and flag or triage suspected frauds early on based on customers’ raised claim data.
So, those flagged claims are sent to the concerned department for further investigation, leading them to avoid paying for such fraudulent claims and save money on that. In extreme cases, it even enables them to report or stop that customer from performing such actions.
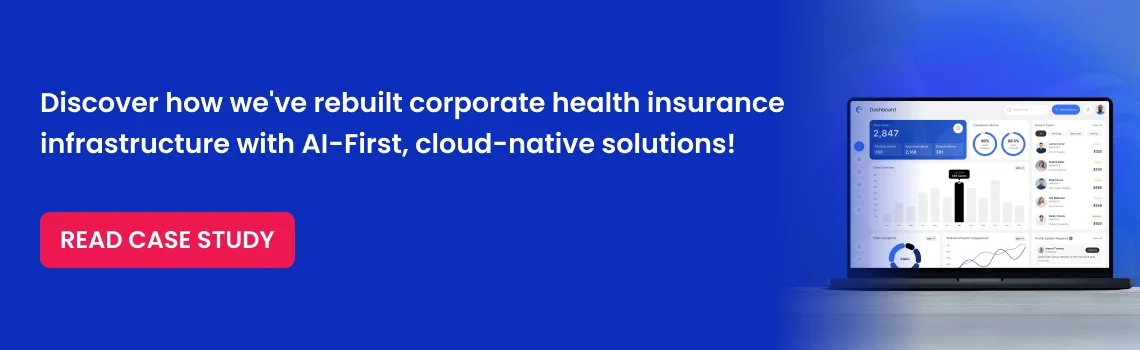
4. Pricing and Premium Optimization
Most of the pricing and premium optimization for insurance is done based on their claims history. However, predictive analytics can help to optimize insurance pricing and premiums even more accurately without excessive manual intervention.
So how is predictive analytics used in insurance pricing and premium optimization? It does that by undertaking assessments of market trends, customer behavior, associated risk factors, claims data, and more to create dynamic pricing strategies while ensuring profitability and customer satisfaction.
5. Customer Churn Prediction and Retention
The insurance industry is highly competitive, where the majority of customers prefer to switch to another insurance provider that offers them competitive pricing and benefits. This is very much relevant for auto insurance companies, specifically. Hence, it becomes essential to identify customers who are at risk of discontinuing their policies.
Predictive analytics provide insurers with data about churn rate by analyzing engagement metrics and satisfaction levels. These insights allow insurers to implement targeted retention strategies, such as personalized offers or improved services, to retain valuable customers.
Steps to Implement Predictive Analytics in Insurance
Implementing predictive analytics for insurance industry operations requires a strategic approach, which includes defining business objectives, collecting and integrating relevant data, data preprocessing and feature engineering, model selection, model training and validation, and complying with industry regulatory standards.
Below, we are going to provide a detailed breakdown of a step-by-step process to implement predictive analytics in insurance products:
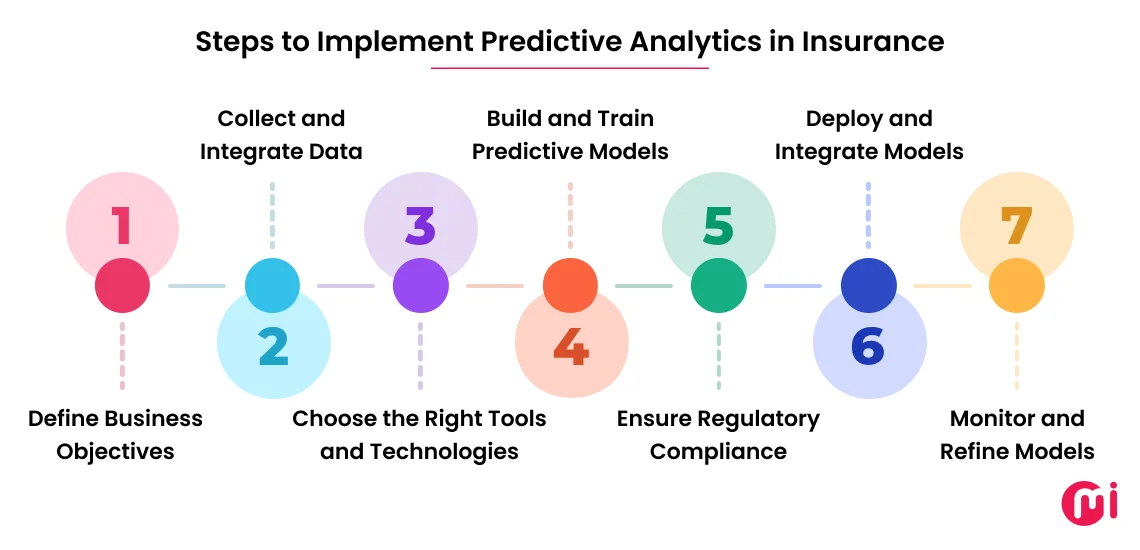
STEP 1: Define Business Objectives
- Collaborate with actuaries, underwriters, claims managers, and executives to determine specific objectives and set KPIs.
STEP 2: Collect and Integrate Data
- Gather internal data, including policyholder data (demographics, policy details, payment history), claims data (past claims, types, severity, resolution time), and underwriting data (risk profiles, premiums, coverage details).
- Also, ingest external data, such as credit scores, driving records, or social media activity (where legally permissible); telematics data for auto insurance; or weather or geographic data for property insurance.
- Clean data to remove duplicates, correct errors, and handle missing values.
- Use data warehouses or platforms like Snowflake, Hadoop, or cloud-based solutions (AWS, Azure) to centralize structured and unstructured data.
Want to know more about data warehouses? Read our blog on data lakes vs. data warehouses vs. data marts.
STEP 3: Choose the Right Tools and Technologies
- Select analytics platforms and tools like Snowflake, Databricks, Apache Spark, Apache Airflow, Docker, PySpark, Grafana Labs, Datadog, Gemini, Apache Kafka, and PubSub.
- Use machine learning frameworks, like TensorFlow, PyTorch, or cloud-based ML platforms (Google AI, Azure ML).
- Use data visualization tools like Tableau, Looker, Power BI, or others for reporting and dashboards.
- Leverage scalable cloud platforms, like AWS, Google Cloud, Microsoft Azure, or Digital Ocean.
- Integrate predictive models into existing systems via APIs for real-time decision-making.
STEP 4: Build and Train Predictive Models
- Select the right use cases from risk assessment, fraud detection, customer segmentation, churn prediction, or something else, or all.
- Choose algorithms like regression models (for pricing and risk scoring), decision trees, random forests, or gradient boosting (for classification tasks), neural networks for complex patterns, or clustering algorithms like K-means (for customer segmentation).
- Create relevant features, such as claim frequency, policy tenure, or geographic risk scores.
- Split data into training, validation, and test sets to ensure model accuracy and avoid overfitting.
STEP 5: Ensure Regulatory Compliance
- Adhere to data privacy laws like GDPR, CCPA, or HIPAA when dealing with personal data.
- Regularly audit models to avoid predictive bias on race, gender, or other protected attributes.
- Use interpretable models or techniques like SHAP/LIME to explain predictions to regulators and customers.
- Document processes, like data usage, model development, and validation for regulatory audits.
STEP 6: Deploy and Integrate Models
- Integrate predictive models into existing workflows.
- Embed models into real-time systems for tasks like dynamic pricing or automated claims triage.
- Continuously track models’ accuracy, drift, and business impact using metrics like precision, recall, or ROI.
STEP 7: Monitor and Refine Models
- Use the dashboard to continuously monitor KPIs and model performance in real-time and retrain models periodically on new data to refine them to meet changing customer behaviors or market conditions.
- Also, use insights from claims adjusters, underwriters, or customers to improve model accuracy.
Challenges in Introducing Predictive Analytics in Insurance Operations
When implementing or introducing predictive models in insurance operations, you can face challenges like data quality and availability, legacy IT infrastructure, model bias and fairness issues, lack of skilled talent, resistance to change, and even data privacy and regulatory compliance.
Let’s check out the most common challenges insurers face when implementing predictive analytics in their insurance operations with solutions:
1. Data Silos
The challenge:
Insurance companies are designed differently from other ones as they have many channel partners. They are external entities, like insurance agents, brokers, or other intermediaries, that help an insurance company sell and distribute its products and services to customers.
Plus, they have fragmented legacy systems for underwriting, claims, customer services, and actuarial departments. All these departments store data in fragmented systems, resulting in limited visibility and a lack of unified insights.
Issues it creates:
- Incomplete or inconsistent data
- Slower model training due to fragmented access
- Inaccurate predictions due to partial information
- Difficulties in cross-departmental collaboration
Solutions:
- Implement centralized data warehouses or data lakes.
- Use data integration tools (ETL pipelines and APIs) and modern techniques to unify and modernize legacy systems.
- Promote a data governance strategy that ensures consistency, security, and accessibility across departments.
- Employ master data management (MDM) to create a single source of truth.
Speaking of which, our big data services can add significant value to help you deal with this challenge.
2. Talent Gap
The challenge:
The insurance industry is full of sales agents, legal teams, and underwriters. This makes it very obvious for them to have a shortage of skilled professionals who possess both domain knowledge of insurance and technical expertise in data science, machine learning, and AI.
Issues it creates:
- Misalignment between business goals and model outcomes
- Inability to operationalize or scale predictive models
- Heavy reliance on external consultants, which increases cost and reduces agility
Solutions:
- Upskilling the existing team through training programs in data science and AI.
- Hire data engineers and data analysts to act as extended teams, bridging the gap between analytics and insurance experience.
3. Regulatory Hurdles
The challenge:
The insurance industry is highly regulated (with standards like IFRS, FSAP, or others). When you opt for AI integration services to enable smart capabilities for decision-making, especially in underwriting or claims, it’s a must to comply with strict fairness, transparency, and auditability standards. That’s a big hurdle for the insurance industry to comply with.
Issues it creates:
- Models must be explainable and auditable
- Restrictions on using certain types of data (e.g., credit scores, health records)
- Increased risk of legal repercussions if bias or discrimination is detected
Solutions:
- Leverage AI development services to implement explainable AI frameworks (e.g., SHAP, LIME) to make model decisions interpretable.
- Align model design with industry-specific regulations like GDPR, HIPAA, and state insurance laws.
- Include compliance and legal experts early in the model development lifecycle.
- Document all assumptions, variables, and outcomes for regulatory audits.
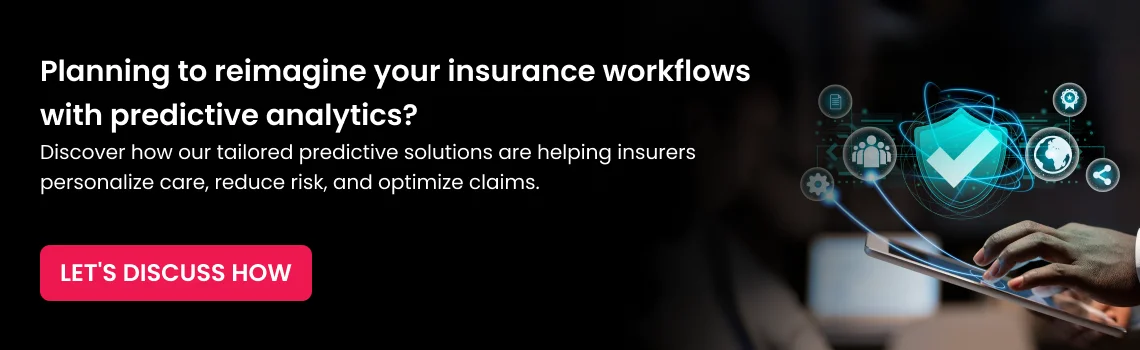
Change Management Strategies With Predictive Model Implementation
To successfully implement predictive analytics in insurance industry operations with change management, it’s a must to focus on clear communication, proactive engagement, and a structured approach.
Best change management strategies with the implementation of predictive analytics for insurance include:
- Leadership Buy-In: Securing commitment from senior executives and department heads by presenting an existing business case focused on ROI and success stories from industry peers to make its adoption easygoing across the organization.
- Employee Training & Upskilling: Educating and empowering teams (underwriters, claims managers, actuaries, etc.) to work confidently with predictive models and trust their outcomes while demystifying the “black box” AI/ML tech term.
- Phased Rollout: Start small with a pilot-first approach to implement predictive models in low-risk environments or specific teams before scaling across the organization to build trust.
- Stakeholder Communication: Maintaining transparent, ongoing communication with all parties impacted by predictive analytics across business units, compliance, IT, and customers.
Along with this, industry leaders also like to read our AI strategy implementation guide.
Real World Examples of Predictive Analytics in Insurance
The real-world examples of predictive analytics in insurance are mainly around identifying high-risk customers, detecting fraud, optimizing underwriting and pricing, and improving claims management cycles. But are there any insurance companies currently leveraging predictive analytics in their workflows?
Well, yes! Let’s discuss real-world examples of the use of predictive analytics in industry businesses:
#1 Progressive – Snapshot® Program
Progressive is a leading American insurance company, serving 18 million customers with various products for cars, homes, motorcycles, RVs, and boats. For the auto insurance segment, it runs a revolutionary Snapshot Program, in which it attaches a small electronic device to customers’ cars to track telematics data, including customers’ driving habits, hard braking, total mileage, and car driving time data.
Leveraging this data, its predictive model and analytics processes help them fine-tune policy pricing. This use of predictive analytics in the auto insurance segment not only helps Progressive Insurance Company to make more profit but also enables 1.4 million drivers enrolled to get premium discounts.
#2 John Hancock
John Hancock Financial is a life insurance company based in Boston, USA. It has expanded its data analytics capabilities to use predictive analytics in the life insurance segment to help their customers enrolled under John Hancock Retirement Plan Services (JHPS) to save more for retirement.
It does that by analyzing their customer data, specifically for defined contribution (DC) plans, to know why they are taking loans on it, what they were doing before taking loans, and what they’ll do post that (is it for buying a house, tuition fees, or something else?). The use of predictive analytics helps them segment customers to offer better, optimized solutions for financing activities.
Apart from that, many other insurance firms, like MetLife, AXA Climate, and more, are also leveraging predictive analytics in their operations for better insurance business benefits.
Future Outlook (2025-2030): Trends Revolutionizing Predictive Analytics In Insurance
The future of predictive analytics in insurance seems brighter in leveraging data to hyper-personalize products/services’ offerings and enhance customer experiences.
Let’s check out future trends in predictive analytics for the insurance industry:
- Achieving hyper-personalization where real-time predictive analytics helps to deliver highly customized products, pricing, and communication tailored to each customer. For example, auto insurers will adjust premiums in real time based on driving patterns captured by telematics. Health insurers will design wellness programs tailored to individual fitness tracker data. Customers will receive dynamic policy recommendations (e.g., travel insurance triggered by flight bookings).
- Leveraging ethical AI in predictive model training to mitigate bias, achieve better explainability (using tools like SHAP and LIME), and align with growing AI governance standards (e.g., ISO 42001, EU AI Act, or NAIC model laws).
- Embracing embedded insurance integrated into customer lifestyle via API-driven ecosystems. It will empower predictive analytics for real-time understanding with contextual understanding, data-driven enablement for micro-policy creation, and enhanced customer segmentation. The best example includes predicting the need for travel insurance based on historical booking behavior and offering embedded coverage in real time.
- AI-driven underwriting 2.0 that will shift from periodic assessment to continuous risk monitoring. For example, real-time health data from wearables will adjust life insurance premiums. Property insurers will use satellite imagery and climate models to update coverage for climate-vulnerable areas.
- The use of generative AI in insurance will unlock predictive scenario modeling. It includes simulating catastrophic events (e.g., hurricanes) to optimize reinsurance strategies. It will also help to generate synthetic data to fill gaps to improve predictive model accuracy and automate claims assessments, like flagging fraudulent claims.
Ready to Transform Your Insurance Business with Predictive Analytics? Do it with MindInventory!
The future of insurance belongs to those who act today. With AI becoming a necessity today, predictive analytics has evolved into more than just a tool for forecasting risks. Today, it’s redefining customer experiences, streamlining operations, and helping insurance companies stay ahead in a hyper-competitive market.
To implement and make the most of predictive analytics in your insurance workflows, you need tech expertise. That’s where MindInventory comes in as your reliable data science services company, helping you in implementing predictive analytics across your workflows.
Here’s what you’ll benefit from partnering with MindInventory:
- Industry-specific expertise and experience working on diverse insurance projects – from health insurance to P&C insurance firms.
- End-to-end implementation support, from data integration and model development to compliance and change management.
- Expertise in building ethical AI frameworks that align with global regulations (ISO 42001)
- Future-proofing analytics with cloud engineering services and generative AI services.
What will be our next step?
- Audit your existing data ecosystem and identify high-impact use cases.
- Start small with implementing a low-risk pilot project (e.g., claims triage or churn prediction) and measure ROI in 12 weeks.
- Deploy enterprise-wide predictive workflows with continuous optimization.
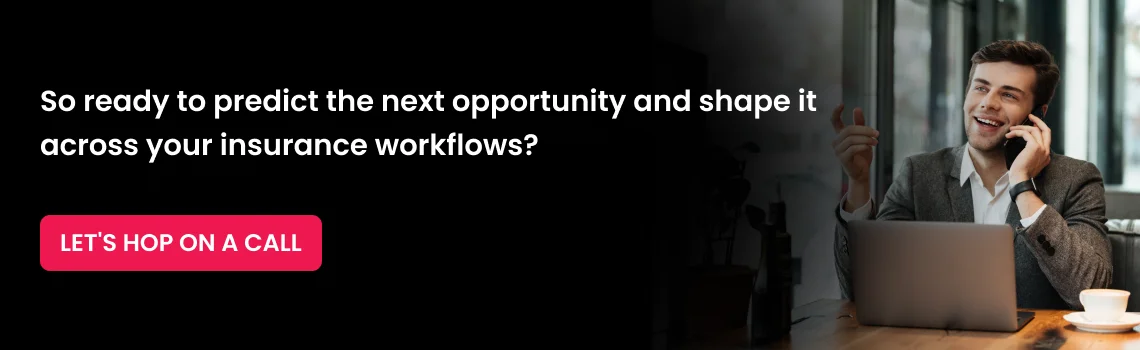
FAQs About Predictive Analytics in Insurance
Predictive analytics detects insurance fraud by analyzing historical claims, identifying unusual patterns, and flagging high-risk activities early, especially at the FNOL (First Notice of Loss) stage, which leads to faster, more accurate fraud prevention.
You can expect the cost to implement predictive analytics in insurance to be somewhere in the range of $50,000 to $500,000+. It can also vary depending on factors like project scope, data infrastructure, model complexity, vendor involvement, ongoing maintenance, and the cost to hire data and AI talents.
It may take from a few weeks to months to implement predictive analytics in an insurance platform. The timeline also differs depending on factors like data readiness, technology maturity, integration complexity, and internal buy-in.
Key risks include biased data, lack of transparency (“black-box” AI), regulatory non-compliance, and customer mistrust if predictive decisions are not explainable or fair.
AI enhances underwriting by analyzing massive datasets to assess risk, recommend coverage, and price policies accurately. This leads to faster decision-making, fewer manual errors, and hyper-personalized offers.
Predictive analytics is used in insurance to forecast claims, detect fraud, assess risk, optimize pricing, and improve customer retention through churn prediction and personalized outreach.
You can measure the ROI of predictive analytics in insurance by considering three main metrics. — — Metric 1: Time-to-decision reduction;
– Metric 2: Fraud detection false positives/negatives ratio;
– Metric 3: Employee productivity gains.
Apart from that, you can also consider faster claims resolution, fraud detection accuracy, policyholder retention, premium uplift, and employee productivity improvements for further ROI measurements.
Traditional analytics explains past events, while predictive analytics forecasts future outcomes using machine learning, enabling insurers to make proactive, real-time decisions.